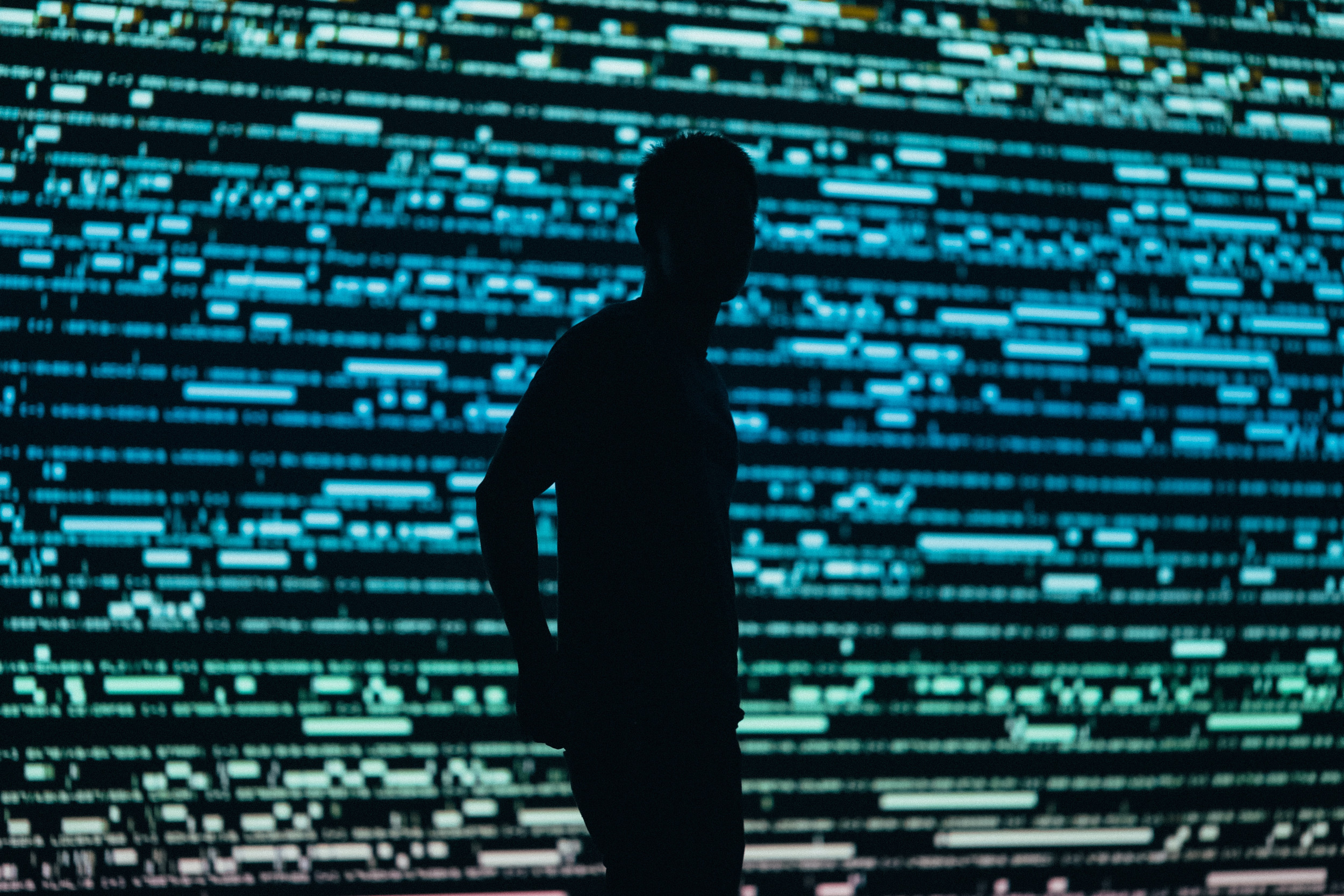
Photo by Chris Yang on Unsplash
Start date: 1st August 2022
End date: 30th July 2025
Funding agency: US European Office of Aerospace Research and Development
Abstract
NS-CEP will investigate uncertainty-aware neuro-symbolic architectures for maintaining constant situational understanding by autonomously identifying novel classes of complex events in a high speed, large bandwidth, signal sensor network, like in modern cyber-physical systems. This project will improve the state-of-the-art by going beyond the current paradigm that heavily relies on neural networks for summarising high-dimensional data for further analysis with symbolic mechanisms. We propose to overcome this dichotomy using leading the research agenda in the ever-growing field of neuro-symbolic/neural-algorithmic reasoning.
Future military operations will span multiple domains and timeframes and require highly agile integrated systems that draw on the strengths of human and AI agents working together. MDO describes how a military force, as part of a joint force operation, must counter and defeat a near-peer adversary capable of contesting in all domains, from competition to armed conflict, in the 2025-2050 timeframe. Such capabilities require constant situational understanding of yet unforeseen complex events in high speed, large bandwidth, signal sensor networks, to ensure adversary activities are understood and countered in effective and proportionate ways. NS-CEP will offer responsive capabilities to inform decision-making and provide wide-ranging situational understanding in pre-conflict, conflict and post-conflict situations.
NS-CEP will investigate efficient engineering of novel uncertainty-aware neuro-symbolic complex event processing for situational understanding in MDO. In particular, we will work towards the following objectives:
- to derive tensorised versions of arbitrary probabilistic circuits that can thus be implemented using standard programming libraries such as Tensorflow and PyTorch in order to embed logical statements in neuro-symbolic architectures efficiently;
- to equip our neuro-symbolic architecture for complex event processing with approximated Bayesian techniques for uncertainty-aware learning and reasoning in order to enable the ability to identify as novel unseen complex events;
- to test the proposed approaches against widely recognised datasets or datasets specifically designed by us, thus triggering additional interest in the community in order to mature industry-ready techniques at technology readiness level 4provide empirical evidence of efficiency.
We will build on top of recent advancements in situational understanding that emerged from the DAIS-ITA project—cofunded by the US CCDC ARL and the UK Dstl—notably the research in evidential learning and reasoning and on complex event processing. NS-CEP will also leverage synergies of a long-lasting collaboration with UCLA, that is submitting another application the same funding call. UCLA’s proposal will complement NS-CEP by focusing on uncertainty-aware space-time relationships between simple and complex events, identifying the boundaries between the neural and the symbolic parts—the bottleneck—and operating on a fully distributed paradigm.