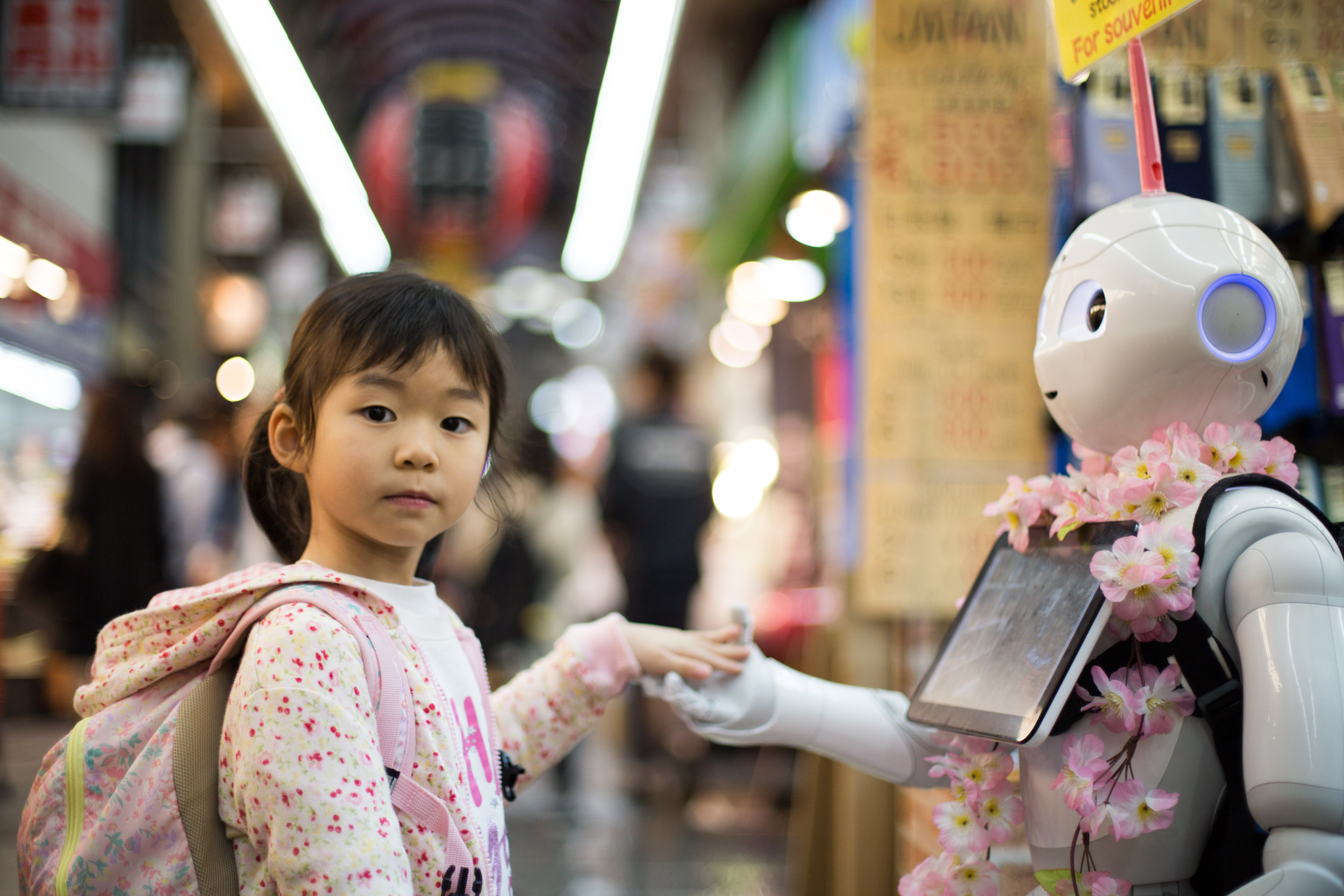
Photo by Andy Kelly on Unsplash
Start date: 1st October 2022
End date: 30th September 2024
Funding agency: USA Devcom ARL
In collaboration with:
- UCLA, University of California Los Angeles, USA (Leader)
- Cardiff University, UK
- Imperial College London, UK
Abstract
Embodied AI-enabled agents are critical in extreme, highly dynamic, and contested environments. Joint All-Domain Operations depend on efficiently coordinating and synchronizing perception, cognition, communication, and actions among agents at the tactical edge and between the edge and command-control. This project aims to overcome current barriers to AI working effectively with humans. It will enable teams of humans and AI agents to collaborate effectively, where agents learn, both during a mission and from one mission to another, how to efficiently coordinate their local perception-cognition-communication-action (PCCA) loops with team-wide global PCCA loops.
The project team — a UCLA-led partnership that also engages researchers from the Cardiff University (UK), the Imperial College (UK), and the University of Brescia (Italy) along with collaborators from the Army Research Laboratory — adopts an approach that is inspired by their research under the now completed US-UK DAIS ITA program (https://dais-legacy.org, 2016-2021). That research focused on explainable and uncertainty-aware AI/ML-enabled systems with neurosymbolic architectures that facilitate trust calibration, allow for the injection of human knowledge and provide robustness to adversaries and domain shifts.
The project’s approach to coordinating physical and informational actions in human-AI teams is one of multi-objective neurosymbolic reinforcement learning (RL). Within that broad framework, the primary intellectual merit elements of the project consist of:
- A hierarchical neurosymbolic RL framework that would allow collaborating AI agents in a team to independently learn adaptable local policies, specific to their skills and abilities while taking into account and deciding upon, communications with other AI agents and humans.
- Methods for distributed orchestration across the human-AI team of cyber-physical actions whose quality of result and probability of success depend on the network and spatiotemporal context of the human or the AI agent.
- Robust, agile, and general methods that allow neurosymbolic AI systems to provide humans with appropriate explanations (appropriate in form, circumstances, and recipient) accompanying information that is explicitly or implicitly communicated to the humans, and assimilate new knowledge received from humans via either explicitly communicated messages or indirect observations of cyber-physical actions.
- A distributed uncertainty measurement, calibration, and propagation framework to enable AI agents to adapt RL policies in response to operational dynamics, domain shifts, and new tactics, techniques, and procedures.
The project team will validate its research through physics-based emulations with virtual AI-enabled and human agents, and sub-scale real-life experiments using small teams of robotic platforms and humans in emulated scenarios.
The anticipated outcomes are:
- Analytical and empirical characterizations of the distributed hierarchical neurosymbolic RL framework, the methods for explanation and knowledge injection, and uncertainty-driven adaptation.
- Algorithms for the neurosymbolic RL framework to take actions and learn efficiently, in real-time, and robustly in distributed, adversarial, and uncertain settings.
- Open-source software and synthetic data sets to enable research validation and reproducibility.
The project will lead to new architectures for teams of embodied AI agents and humans to robustly perform missions involving diverse information and physical actions, and rapidly adapt to novel settings.